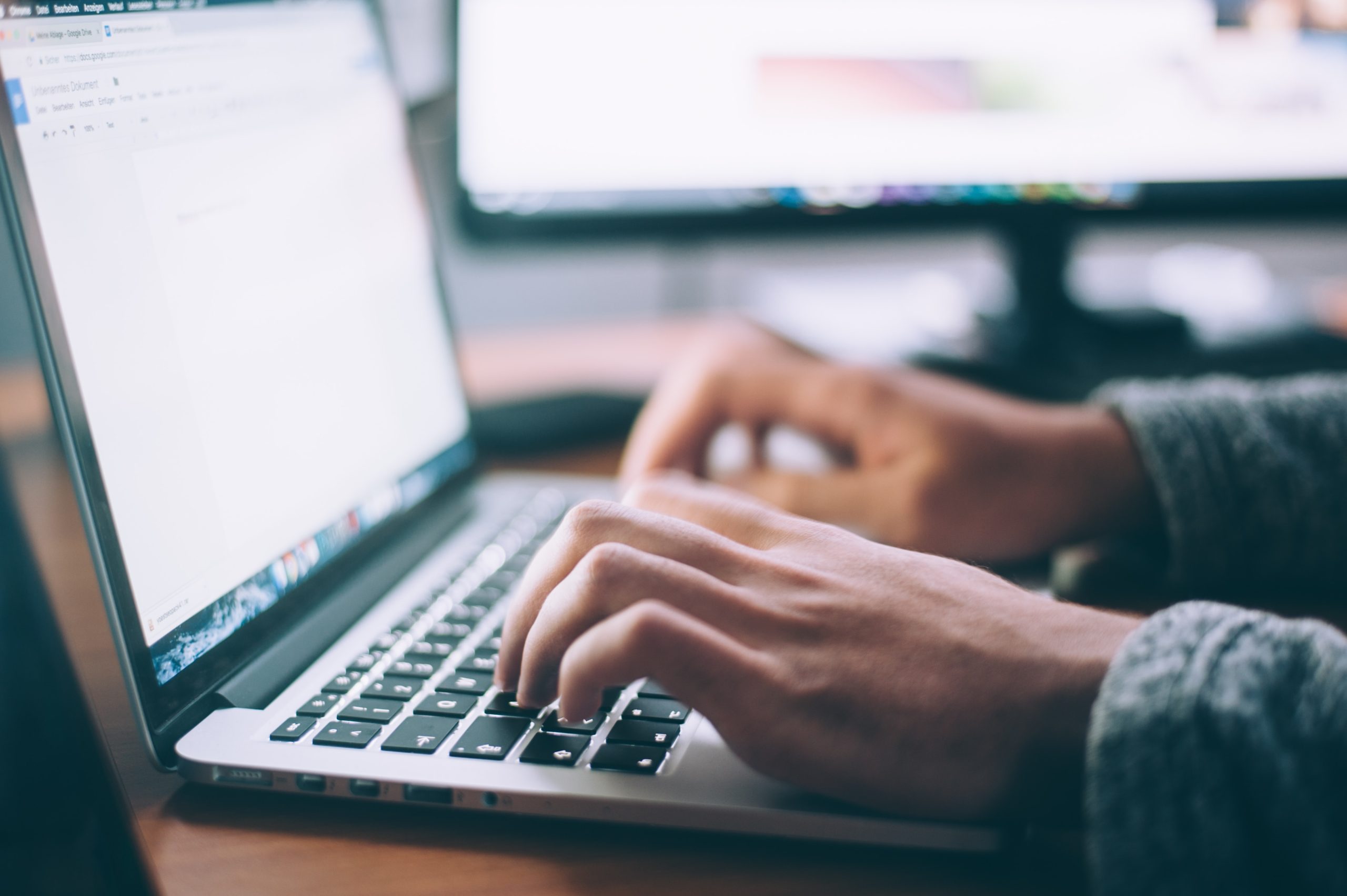
Unearthing new drugs to combat diseases has always been a complex and time-consuming process. However, with the advent of artificial intelligence (AI), drug design is undergoing a revolutionary transformation. AI-driven molecular docking, in particular, is emerging as a game-changer in the field of pharmaceutical research.
Imagine a world where scientists can accurately predict how different molecules will interact with target proteins, speeding up the discovery of life-saving medications. This groundbreaking approach harnesses the power of AI algorithms to simulate and analyze millions of potential drug candidates, significantly reducing both cost and time required for traditional trial-and-error methods.
In this blog post, we will explore the fascinating realm of AI-driven molecular docking and its profound impact on drug design. From understanding its role in revolutionizing pharmaceutical research to uncovering real-life success stories, we’ll delve into the benefits and challenges associated with this cutting-edge technology. So fasten your seatbelts as we embark on an exciting journey at the intersection of science and innovation!
The Role of Artificial Intelligence in Drug Design
Artificial Intelligence (AI) has emerged as a game-changer in various fields, and drug design is no exception. The role of AI in drug design has revolutionized the way scientists approach the discovery of new drugs. With its ability to analyze vast amounts of data quickly and accurately, AI has become an indispensable tool in molecular docking.
Molecular docking plays a crucial role in understanding how potential drug molecules interact with target proteins or receptors. It helps researchers identify promising compounds that have the potential to bind effectively to these targets and elicit a desired therapeutic response.
AI-driven molecular docking takes this process to the next level by leveraging machine learning algorithms and predictive modeling techniques. By training AI models on large databases of known interactions between drugs and target proteins, scientists can predict with high accuracy which compound is most likely to bind effectively.
The benefits of using AI-driven molecular docking are numerous. It significantly speeds up the drug discovery process by rapidly narrowing down the pool of potential candidates for further testing. This enables researchers to focus their efforts on compounds that are more likely to succeed, saving valuable time and resources.
AI-driven approaches enhance efficiency by reducing costly trial-and-error experiments often associated with traditional methods. By relying on computational predictions generated by AI models, researchers can prioritize experimental validation only for those compounds that show significant promise.
AI allows for greater exploration of chemical space and identification of novel scaffolds that may not have been considered using conventional methods alone. This expands the possibilities for finding innovative drugs targeting complex diseases where existing treatments fall short.
Several successful case studies demonstrate how AI-driven molecular docking has led to groundbreaking discoveries in drug design. For instance, deep learning algorithms have aided in identifying potential inhibitors for HIV protease enzymes – essential targets in developing antiretroviral therapies against AIDS.
In another notable example, AI-powered virtual screening identified new small molecules capable of inhibiting cancer-related protein kinases involved in tumor growth pathways.
Benefits of AI-Driven Molecular Docking
AI-driven molecular docking has revolutionized the field of drug design by offering numerous benefits. One major advantage is the speed and efficiency with which AI algorithms can analyze vast amounts of data and generate potential drug candidates. Traditional methods for molecular docking required manual input and were time-consuming, but AI-powered systems can rapidly screen thousands or even millions of compounds to identify those with the highest binding affinity to a target protein.
Another benefit is the increased accuracy and precision achieved through AI-driven approaches. By utilizing machine learning algorithms, these systems can continuously learn from previous docking experiments and improve their performance over time. This means that as more data becomes available, AI models become increasingly adept at predicting how different molecules will interact with specific proteins.
AI-driven molecular docking enables researchers to explore a wider range of chemical space in search of new drug candidates. Traditional methods often focused on known chemical scaffolds or analogs, but AI models have the ability to suggest novel compound structures that may exhibit better pharmacological properties.
Using AI for molecular docking helps reduce costs associated with experimental testing. By employing virtual screening techniques early in the drug discovery process, researchers can prioritize promising compounds for further evaluation instead of wasting resources on synthesizing and testing large numbers of potentially ineffective or unsafe molecules.
This technology also allows for personalized medicine approaches by considering individual patient characteristics such as genetic variations or specific disease subtypes when designing drugs.
The benefits derived from using artificial intelligence in molecular docking are numerous: increased speed and efficiency, improved accuracy and precision, exploration of novel chemical space, reduced costs through virtual screening, and potential for personalized medicine applications.
Successful Drug Discoveries Using AI-Driven Molecular Docking
In recent years, the integration of artificial intelligence (AI) into the field of drug design has revolutionized the way scientists approach molecular docking. By harnessing the power of AI, researchers are able to streamline and accelerate the process of discovering potential drug candidates. Let’s take a look at some notable case studies where AI-driven molecular docking has led to successful drug discoveries.
One such example is the discovery of a promising anti-cancer drug called Venetoclax. Using AI-driven molecular docking algorithms, scientists were able to identify a small molecule that specifically targets and inhibits B-cell lymphoma 2 (BCL-2), a protein known for promoting cancer cell survival. This breakthrough has paved the way for more effective treatments in various types of cancers.
Another remarkable case involves the development of Osimertinib, an FDA-approved medication used to treat non-small cell lung cancer with specific genetic mutations. Through AI-driven molecular docking techniques, researchers were able to identify Osimertinib as a potent inhibitor against epidermal growth factor receptor (EGFR) mutations commonly found in lung cancer patients.
AI-based tools have also played a pivotal role in combating infectious diseases like COVID-19. Researchers utilized molecular docking simulations powered by machine learning algorithms to screen millions of compounds and identify potential drugs that could inhibit key proteins essential for viral replication.
These case studies highlight just a few instances where AI-driven molecular docking has made significant contributions to drug discovery efforts. The ability to rapidly screen vast libraries of molecules and predict their interactions with target proteins allows scientists to optimize lead compounds efficiently and expedite promising candidates through preclinical and clinical trials.
The success stories mentioned above demonstrate how integrating AI into traditional drug design approaches can greatly enhance efficiency while reducing costs and time required for development. As technology continues advancing at an unprecedented pace, we can expect even more groundbreaking discoveries and advancements in the field of drug design through AI-driven molecular docking.
Challenges and Limitations of AI-Driven Molecular Docking
Despite its promising potential, AI-driven molecular docking also faces several challenges and limitations. One major challenge is the accuracy of the generated models. While AI algorithms can process vast amounts of data and make predictions, there is always a possibility for errors or inaccuracies in the results. It is crucial to validate these models through experimental testing to ensure their reliability.
Another limitation lies in the availability and quality of data. AI algorithms heavily rely on large datasets for training, but obtaining comprehensive and diverse datasets can be a challenge in drug design. Moreover, the quality of available data may vary, leading to biased or incomplete predictive models.
Interpreting and understanding the output generated by AI systems can be complex. The black-box nature of some AI algorithms makes it difficult to explain how decisions are made or identify potential biases within the system.
Computational power plays a significant role in implementing complex AI-driven molecular docking techniques. High-performance computing resources are required to handle large-scale calculations effectively.
Ethical considerations surrounding privacy and security must also be addressed when using AI-driven methods in drug design. Protecting patient information and ensuring ethical use of data are essential aspects that need careful attention.
Overcoming these challenges will require continued research, collaboration between experts from different fields (such as computer science, chemistry, biology), advancements in technology infrastructure (including improved hardware capabilities), enhanced data sharing mechanisms while safeguarding privacy rights, and transparent reporting standards for accountability.
By acknowledging these challenges upfront and working towards innovative solutions collectively, we can harness the true potential of AI-driven molecular docking techniques in revolutionizing drug design processes.
The Future of Drug Design with AI
As technology continues to advance at an exponential rate, the future of drug design looks incredibly promising with the integration of artificial intelligence (AI). AI-driven molecular docking has already demonstrated its potential in revolutionizing the way drugs are discovered and developed.
One key aspect that holds great promise for the future is the ability of AI systems to quickly analyze vast amounts of data. With this capability, researchers can efficiently sift through a multitude of molecules and predict their interactions with target proteins or receptors. This significantly accelerates the drug discovery process, potentially leading to faster development of new treatments.
AI algorithms can learn from previous successful drug designs and use this knowledge to generate novel compounds. By leveraging existing data on known drugs and their mechanisms of action, AI can propose innovative molecules that may have never been considered before by human researchers. This opens up exciting possibilities for finding entirely new classes of therapeutics.
In addition to speeding up drug discovery, AI also has immense potential in optimizing existing drugs. By simulating how different modifications impact a molecule’s properties or efficacy, researchers can tailor these compounds to specific needs more effectively than ever before.
Another area where AI shows promise is in personalized medicine. By analyzing individual patients’ genetic information and medical history, algorithms could identify optimal treatment options tailored specifically for each person’s unique characteristics.
It is important to recognize that there are still challenges and limitations ahead when it comes to fully harnessing the power of AI in drug design. The reliability and interpretability issues associated with some machine learning models need to be addressed so that decision-making processes are transparent and reliable.
Conclusion
The integration of artificial intelligence into molecular docking has revolutionized the field of drug design. This powerful combination has significantly accelerated the process of identifying potential therapeutic compounds and optimizing their interactions with target proteins.
AI-driven molecular docking offers numerous benefits, including increased speed and efficiency in screening large compound libraries, enhanced accuracy in predicting binding affinities, and improved understanding of complex protein-ligand interactions. These advancements have led to successful drug discoveries across various disease areas.
Although AI-driven molecular docking shows immense promise, it is not without its challenges and limitations. The need for high-quality training data, potential biases in algorithmic predictions, and computational resource requirements are some hurdles that researchers must overcome. However, ongoing research efforts continue to address these issues and further refine AI-powered drug design techniques.
So let us embrace this exciting era where science fiction becomes reality through AI-empowered drug discovery methods – a journey towards a healthier tomorrow!