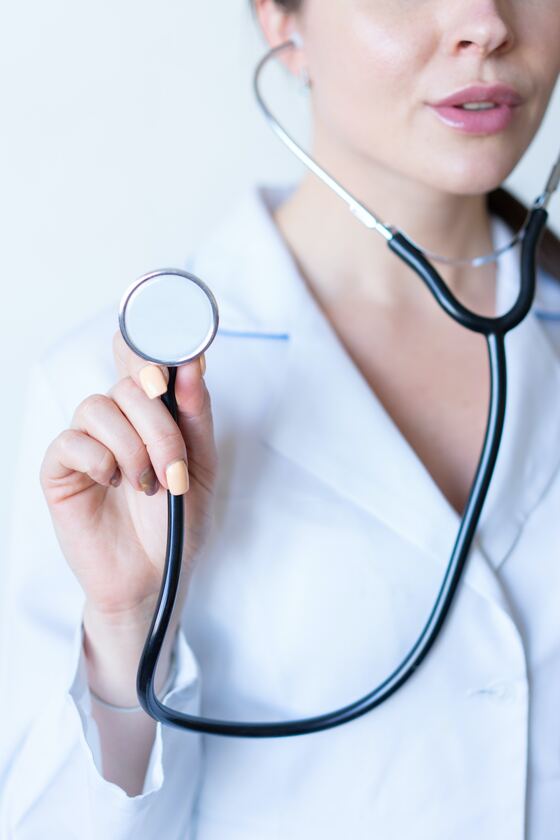
Are you ready to unlock the power of data in trials like never before? Dive into the world of Machine Learning and Predictive Analysis with us as we explore how this cutting-edge technology is revolutionizing the way we interpret trial data. Get ready to discover the endless possibilities that come with leveraging machine learning for predictive analysis in trials!
Benefits of Using Machine Learning in Trial Data Analysis
Machine Learning offers a multitude of benefits when it comes to analyzing trial data. One major advantage is the ability to identify patterns and trends that may not be easily discernible through traditional methods. By leveraging Machine Learning algorithms, researchers can extract valuable insights from large datasets efficiently.
Machine Learning can enhance predictive analytics in trials by forecasting outcomes based on historical data. This predictive capability enables researchers to make more informed decisions and optimize trial protocols for better results. Additionally, the use of Machine Learning can streamline the data analysis process, saving time and resources while improving accuracy.
Machine Learning models can adapt and learn from new data inputs, continuously refining their predictions over time. This adaptive nature allows for real-time adjustments and improvements in trial strategies based on evolving information. Incorporating Machine Learning into trial data analysis holds immense potential for revolutionizing research methodologies and driving scientific advancements forward.
Types of Predictive Models in Trials
When it comes to predictive models in trials, there are various approaches that can be leveraged to analyze data and make informed decisions. One common type is logistic regression, which predicts the likelihood of a binary outcome based on independent variables. Decision trees use a tree-like graph of decisions and their possible consequences to predict outcomes.
Another popular model is random forest, which builds multiple decision trees and merges them together to improve accuracy. Support Vector Machines (SVM) classify data by finding the hyperplane that best separates different classes. Neural networks mimic the human brain’s structure to learn complex patterns in data for prediction.
Time-series models like ARIMA are used when analyzing sequential data points over time. Ensemble methods combine multiple models for better predictions by averaging or weighting their outputs. Each type of predictive model offers unique strengths and can be tailored to suit specific trial requirements.
Case Studies: Successful Implementation of Machine Learning in Trials
Let’s delve into some real-world applications of machine learning in trials. In a recent study, researchers used predictive modeling to analyze clinical trial data and accurately predict patient responses to new treatments. By leveraging machine learning algorithms, they were able to identify patterns and trends that traditional methods might have missed.
In another case, a pharmaceutical company implemented machine learning techniques to optimize their trial protocols. By analyzing vast amounts of historical data, they were able to streamline the recruitment process for patients matching specific criteria and improve overall trial efficiency.
In the field of personalized medicine, machine learning has been instrumental in tailoring treatment plans based on individual patient profiles. By analyzing genetic data and patient characteristics, healthcare providers can now make more informed decisions regarding treatment options.
These successful implementations highlight the immense potential of machine learning in transforming the landscape of clinical trials.
Challenges and Limitations of Using Machine Learning in Trials
Implementing machine learning in trials comes with its set of challenges and limitations. One major hurdle is the need for high-quality data to train the models accurately. Garbage in, garbage out – as they say. Without clean and relevant data, the predictive analysis can be skewed or inaccurate.
Another challenge is the interpretability of machine learning algorithms. Sometimes these models work like a black box, making it difficult to explain how a particular prediction was made. This lack of transparency can be a concern in legal settings where decisions need to be justified logically.
There are ethical considerations when using machine learning in trials. Bias in the data used for training can lead to biased predictions, potentially perpetuating existing inequalities within the justice system.
Integrating new technology into traditional trial processes may face resistance from stakeholders who are unfamiliar or skeptical about its reliability and validity in legal contexts.
Overcoming these challenges requires collaboration between data scientists, legal experts, and policymakers to ensure that the benefits of machine learning are maximized while mitigating potential risks.
Conclusion
In the rapidly evolving landscape of clinical trials, leveraging machine learning for predictive analysis is proving to be a game-changer. By harnessing the power of data and technology, researchers are able to streamline processes, optimize decision-making, and ultimately improve patient outcomes.
From identifying potential risks and opportunities to enhancing trial design and recruitment strategies, machine learning offers a wealth of benefits for the pharmaceutical industry. The ability to predict outcomes with greater accuracy not only saves time and resources but also holds the promise of bringing life-saving treatments to market faster.
While there are challenges and limitations associated with implementing machine learning in trials, such as data quality issues and regulatory concerns, ongoing advancements in technology continue to address these barriers. As more organizations embrace this cutting-edge approach to data analysis, we can expect even more groundbreaking discoveries on the horizon.
The integration of machine learning into trial data analysis represents a significant step forward in revolutionizing the way we conduct research and develop new therapies. By harnessing predictive models powered by AI algorithms, we have an unprecedented opportunity to unlock insights that could shape the future of healthcare for years to come.