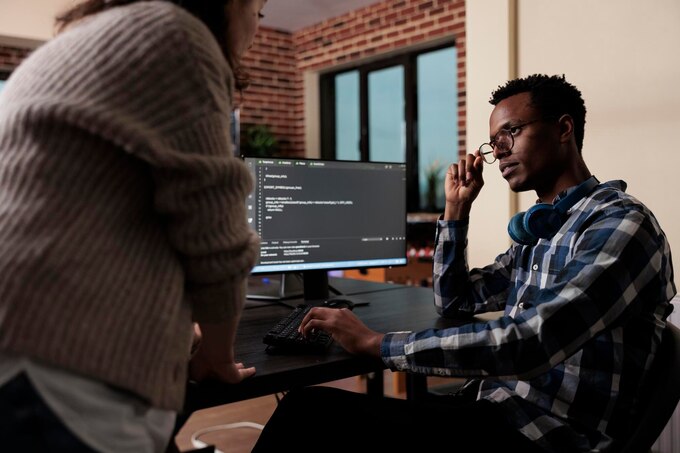
In the world of medical diagnostics, a picture is worth more than a thousand words. It can be the key to understanding complex health issues, leading to better patient outcomes. Multimodal medical imaging takes this concept further by integrating various imaging techniques—such as MRI, CT scans, and ultrasound—to create comprehensive visual representations of a patient’s condition.
But what happens when we introduce artificial intelligence into this mix? Imagine algorithms that not only analyze images but also uncover hidden patterns and insights that even the most experienced radiologists might miss. The fusion of AI with multimodal imaging has the potential to revolutionize healthcare as we know it, bridging gaps in diagnosis and treatment like never before.
The Role of AI in Multimodal Medical Imaging
Artificial Intelligence plays a transformative role in multimodal medical imaging. By integrating data from different imaging modalities, AI enhances diagnostic accuracy and efficiency.
Machine learning algorithms analyze patterns that would be difficult for humans to discern. These models can process images from MRIs, CT scans, and PET scans simultaneously, providing a comprehensive view of the patient’s condition.
AI also assists radiologists by automating routine tasks. This allows healthcare professionals to focus on more complex aspects of their work, improving patient care overall.
Predictive analytics powered by AI can identify potential health issues before they become critical. This proactive approach helps in crafting personalized treatment plans tailored to individual needs.
As technology continues to evolve, the synergy between AI and multimodal imaging holds promise for advancing precision medicine significantly.
Advantages of AI in Multimodal Medical Imaging
AI enhances multimodal medical imaging by improving accuracy. Algorithms can analyze diverse data sources, such as MRI and CT scans, simultaneously. This leads to more precise diagnoses.
Speed is another significant advantage. AI streamlines the imaging process, allowing radiologists to interpret results faster than ever before. Quick analysis means timely interventions for patients.
AI reduces human error in image interpretation. By eliminating biases and inconsistencies often found in manual assessments, it fosters a higher level of confidence in findings.
Machine learning models continuously evolve with new data inputs. As they learn from vast datasets over time, their predictive capabilities improve markedly.
Collaboration across various imaging modalities becomes seamless with AI integration. It bridges gaps between different techniques and provides comprehensive insights into complex medical conditions.
Challenges and Limitations of AI in Multimodal Medical Imaging
Despite its potential, AI in multimodal medical imaging faces significant hurdles. One major challenge lies in data integration. Different imaging modalities produce varying formats and resolutions, complicating the synthesis of information.
Another concern is the quality and quantity of available training data. Many algorithms require vast datasets to learn effectively. However, obtaining diverse and high-quality images can be a daunting task due to privacy issues and limited access.
Interpretability also poses a problem. Clinicians often struggle to understand how AI reaches its conclusions, leading to hesitance in relying solely on these systems for diagnosis or treatment plans.
Regulatory challenges remain prominent. The healthcare landscape is heavily regulated; ensuring that AI tools comply with standards while still offering innovation can slow down advancements significantly. These obstacles highlight the complexity involved in fully harnessing AI’s capabilities within this field.
Current Applications of AI in Multimodal Medical Imaging
AI is revolutionizing multimodal medical imaging across various healthcare settings. One prominent application is in radiology, where algorithms analyze images from CT scans and MRIs to identify anomalies like tumors or fractures more efficiently than traditional methods.
In oncology, AI systems integrate data from different imaging modalities to create comprehensive patient profiles. This aids in personalized treatment plans based on the specific characteristics of a patient’s cancer.
Another exciting application lies in surgical planning. By combining preoperative imaging with real-time intraoperative data, AI assists surgeons in navigating complex procedures with enhanced accuracy.
Telemedicine also benefits significantly from AI’s capabilities, allowing for remote analysis of multiple imaging types. This ensures timely diagnoses even when specialists are not physically present.
Ongoing research aims to improve predictive analytics by merging historical patient data with current imaging results, ultimately enhancing preventative care strategies.
Conclusion
The integration of artificial intelligence in multimodal medical imaging is transforming healthcare. It enhances diagnostic accuracy, streamlines processes, and ultimately improves patient outcomes. As technology continues to evolve, the potential for innovation in this field remains vast.
While there are challenges to address—such as data privacy and algorithm bias—the benefits of AI cannot be overstated. Its capacity to analyze diverse data types fosters a more holistic view of patient health.
Today’s applications showcase how far we’ve come. From identifying tumors earlier than traditional methods to predicting disease progression through combined imaging techniques, the impact is profound.
As research progresses and new methodologies emerge, the bridge between AI and multimodal medical imaging will only grow stronger. This synergy not only holds promise for clinicians but also offers hope for patients seeking timely and accurate diagnoses. The future looks bright in this fascinating intersection of technology and medicine.