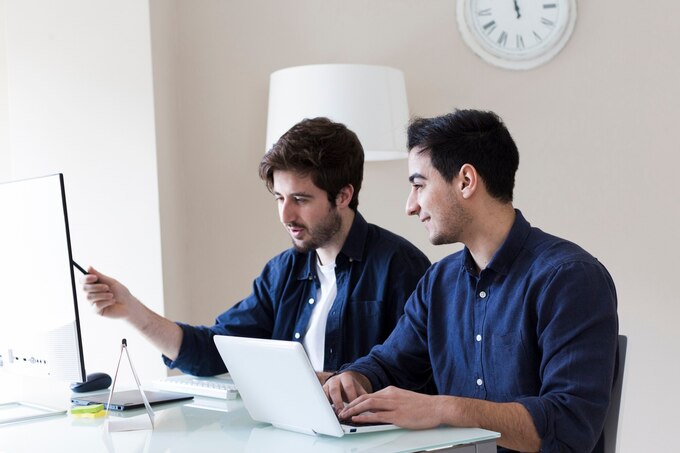
Imagine a world where medical diagnostics are not just reliant on the subjective views of healthcare professionals, but instead backed by the precision and analytical power of artificial intelligence. Welcome to the era of AI-enabled quantitative imaging. This technological advancement is revolutionizing how we visualize and interpret medical data, paving the way for more objective diagnostics.
As healthcare continues to evolve, so does our approach to understanding complex conditions through imaging. Gone are the days when a doctor’s interpretation was solely based on experience and intuition. Today, algorithms trained on vast datasets can assist in detecting nuances that might go unnoticed by even seasoned specialists.
The integration of AI into quantitative imaging brings forth an exciting opportunity: enhanced accuracy, improved patient outcomes, and a transformative shift towards evidence-based medicine. As we delve deeper into this topic, we’ll explore its benefits, applications, and real-world success stories that illustrate how far we’ve come—and where we’re headed next in diagnostic innovation.
Benefits and Applications of AI in Medical Imaging
AI in medical imaging is revolutionizing how we approach diagnostics. With its ability to analyze vast amounts of data, it enhances accuracy and speed in identifying conditions.
One major benefit is early detection. Algorithms can spot anomalies that might be missed by the human eye. This capability can lead to quicker interventions, ultimately improving patient outcomes.
Another advantage lies in workflow efficiency. AI systems assist radiologists by prioritizing cases based on urgency, allowing healthcare professionals to focus on critical patients first.
Moreover, these technologies offer cost-effective solutions for hospitals. By reducing the time needed for diagnosis and minimizing errors, they effectively lower operational costs while maintaining high-quality care.
Applications extend beyond traditional imaging techniques as well. From MRI scans to X-rays and CT images, AI is becoming an invaluable asset across various modalities in medicine.
Overcoming Subjectivity in Traditional Diagnostic Methods
Traditional diagnostic methods often suffer from subjectivity. Radiologists may interpret images differently based on their experience and biases. This inconsistency can lead to misdiagnoses or delayed treatments.
AI-enabled quantitative imaging brings a transformative shift. By using algorithms trained on vast datasets, it establishes standardized metrics for analysis. These metrics provide objective results that reduce the variability seen in human interpretation.
Furthermore, AI systems continuously learn from new data. They adapt over time, improving accuracy as they process more cases. This ongoing evolution means that diagnostics can evolve alongside clinical practices.
By standardizing assessments and minimizing personal bias, AI enhances decision-making in medical contexts. Clinicians can trust these insights to support their judgments better than before.
Case Studies: Real-Life Examples of Successful AI-Enabled Diagnostics
One remarkable case study comes from the partnership between Google Health and radiologists. They developed an AI model to detect breast cancer in mammograms. The technology showed accuracy surpassing human experts by 11%. This innovation is transforming how screenings are approached.
Another compelling example involves a collaboration with Stanford University. Researchers utilized deep learning algorithms to analyze chest X-rays for signs of pneumonia. Their system not only improved diagnosis speed but also reduced error rates significantly, proving invaluable in emergency settings.
In dermatology, a startup created an AI tool that identifies skin conditions through smartphone images. With thousands of cases analyzed, it achieved diagnostic performance comparable to seasoned dermatologists, making expert-level care more accessible than ever.
These examples highlight the potential of AI-enabled diagnostics across various medical fields, opening doors to better patient outcomes and efficiency in healthcare delivery.
Conclusion
The integration of AI-enabled quantitative imaging is reshaping the landscape of medical diagnostics. Its ability to analyze complex data sets quickly and accurately allows for more objective assessments. This shift not only enhances diagnostic precision but also improves patient outcomes.
As we move forward, the importance of leveraging technology in healthcare cannot be overstated. The potential applications are vast, from detecting subtle changes in disease progression to personalizing treatment plans based on precise imaging metrics.
With successful case studies paving the way, it’s clear that this approach holds significant promise for future advancements in medicine. The collaborative efforts between radiologists and AI systems can lead to a new era where decisions are supported by robust data rather than solely relying on subjective interpretations.
Continued research and development will drive improvements in algorithms and methodologies, ensuring that these tools become even more reliable over time. As healthcare professionals embrace this innovation, patients stand to benefit immensely from enhanced accuracy and efficiency in their care journeys.
The future looks bright as AI continues to play an integral role in transforming how we diagnose diseases—making strides towards better health outcomes for everyone involved.