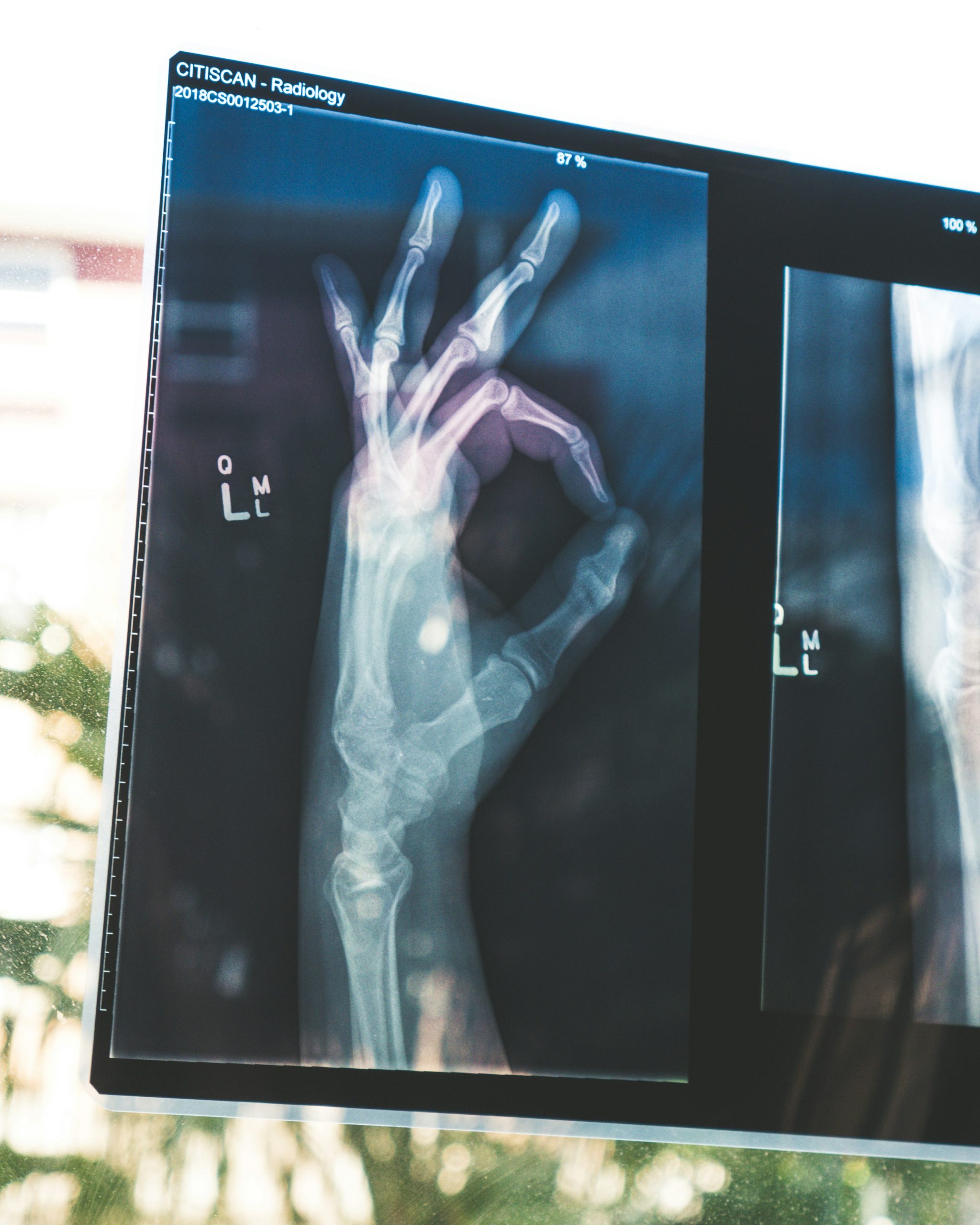
Welcome to a new era in pathology analysis! Gone are the days of manually examining countless slides under a microscope. Thanks to the advancements in machine learning, we now have a powerful tool that is revolutionizing the way pathological analysis is conducted. Machine learning, a branch of artificial intelligence (AI), has found its place in healthcare and is making remarkable contributions to accurate diagnoses and treatment decisions.
In this blog post, we will dive deep into the world of machine learning and explore how it is reshaping pathological analysis. We will uncover its impact on healthcare, discuss the advantages it brings to pathology, highlight some interesting case studies showcasing its success, and ponder upon future possibilities for further advancements. But before we embark on this exciting journey, let’s first understand what machine learning truly entails and how it applies to medicine. So fasten your seatbelts because we’re about to embark on an exhilarating expedition through cutting-edge technology and lifesaving discoveries!
The Impact of Machine Learning on Pathological Analysis
Machine learning has revolutionized many industries, and the field of healthcare is no exception. In particular, it has had a significant impact on pathological analysis. By harnessing the power of algorithms and data analysis, machine learning enables pathologists to make more accurate diagnoses in a shorter amount of time.
One key aspect where machine learning excels is image recognition. Pathological analysis often involves examining tissue samples under a microscope to detect abnormalities or diseases. With machine learning algorithms trained on vast amounts of annotated images, computers can now assist pathologists in identifying patterns and anomalies that may be difficult for the human eye to spot.
Another area where machine learning shines is predictive modeling. By analyzing large datasets containing patient information and medical history, algorithms can predict disease outcomes and treatment responses with remarkable accuracy. This not only aids in personalized medicine but also helps pathologists develop better treatment plans for their patients.
Machine learning has streamlined workflows by automating repetitive tasks such as sorting and organizing data or generating reports. This allows pathologists to focus more on complex cases that require their expertise while reducing errors caused by manual handling.
Despite its numerous advantages, implementing machine learning in pathology does come with challenges. One major hurdle is ensuring the quality and reliability of training data since incorrect or biased annotations could lead to inaccurate results. Additionally, there are concerns about privacy and security when dealing with sensitive patient information.
Looking ahead, there are exciting possibilities for further advancements in this field. As technology continues to improve, we can expect even greater accuracy in diagnoses and predictions through refined algorithms and access to larger datasets.
Advantages of Using Machine Learning in Pathology
Improved accuracy and efficiency: One of the key advantages of using machine learning in pathology is its ability to enhance accuracy and efficiency. Machine learning algorithms can analyze vast amounts of data, enabling pathologists to make more precise diagnoses by identifying patterns that may not be visible to the human eye. This can lead to earlier detection and treatment of diseases, ultimately improving patient outcomes.
Time-saving automation: Pathological analysis often involves time-consuming tasks such as reviewing slides and categorizing tissue samples. Machine learning algorithms can automate these processes, freeing up valuable time for pathologists to focus on complex cases or other important responsibilities. By reducing manual labor, machine learning technology allows for a faster turnaround time in diagnosing patients.
Consistency across diagnoses: Another advantage of machine learning in pathology is the potential for increased consistency across diagnoses. Human error is inevitable, but machines are capable of executing repetitive tasks with extreme precision every single time. By leveraging machine learning algorithms, pathologists can achieve greater standardization in their diagnostic practices, leading to more reliable results.
Enhanced decision support: Machine learning algorithms have the capacity to provide valuable decision support tools for pathologists. They can crunch large datasets containing clinical information and medical imaging findings, assisting pathologists in making more informed decisions about treatment plans or prognoses based on evidence-based recommendations generated by AI models.
Challenges Faced in Implementing Machine Learning in Pathology
1. Data quality and availability: One of the key challenges in implementing machine learning algorithms in pathology is ensuring the quality and availability of data. Pathological analysis requires large amounts of high-quality annotated data to train machine learning models effectively. However, obtaining such datasets can be difficult due to issues like privacy concerns, limited access to patient records, and variations in data collection methods across different healthcare institutions.
2. Lack of standardized protocols: Another challenge lies in the lack of standardized protocols for pathological analysis. Different pathologists may have varying interpretations or approaches when diagnosing diseases, making it challenging to create a uniform dataset for training machine learning models. Developing consensus among experts and establishing standardized guidelines are necessary steps towards overcoming this hurdle.
3. Integration with existing workflows: Integrating machine learning into existing pathology workflows can pose logistical challenges. Pathology laboratories often rely on established processes and systems that may not seamlessly accommodate new technology integration. Adapting workflow patterns and incorporating machine learning tools without disrupting daily operations requires careful planning and coordination.
4. Interpreting complex cases: While machine learning algorithms excel at processing vast amounts of data quickly, they may struggle with interpreting complex cases that require nuanced understanding or expertise beyond pattern recognition alone. Human pathologists possess domain knowledge that allows them to consider various factors before arriving at a diagnosis or treatment plan—an aspect that machines still need refinement on.
5. Technical limitations: The implementation of any new technology comes with technical limitations, including computational power requirements, algorithm complexity, model interpretability, scalability issues, and maintenance costs associated with updating algorithms over time as medical knowledge advances.
As we strive towards harnessing the full potential of machine learning in pathology, it is important to address these challenges diligently through collaborative efforts involving researchers, clinicians, policymakers,and industry stakeholders alike. It is only by doing so that wecan truly unlock the transformative power of this emerging technology in revolutionizing pathological analysis and improving patient outcomes.
Case studies showcasing the success of machine learning in pathology
Case studies showcasing the success of machine learning in pathology have been gaining significant attention in recent years. One notable example is the use of machine learning algorithms to analyze histopathological images for cancer detection. In a study published by researchers at Stanford University, a deep learning model was trained on thousands of labeled images and achieved accuracy levels comparable to human pathologists.
Another case study involves the identification and classification of different types of skin lesions using machine learning techniques. Researchers at Harvard Medical School developed a convolutional neural network that was able to accurately diagnose melanoma with high sensitivity and specificity. This breakthrough has the potential to greatly improve early detection rates and ultimately save lives.
Machine learning has also shown promise in predicting patient outcomes based on molecular profiling data. By analyzing gene expression patterns, researchers have been able to develop predictive models that can help determine which patients are more likely to respond positively or negatively to certain treatments.
Machine learning algorithms have been used successfully in digital pathology workflows for automated tumor grading. These tools aid pathologists by providing objective assessments of tumor characteristics such as size, shape, and cellular features.
Future possibilities and advancements in machine learning for pathological analysis
1. Enhanced Accuracy: One of the key possibilities for machine learning in pathology is its potential to enhance accuracy in diagnosing diseases. Machine learning algorithms can analyze large volumes of medical data, including images and patient records, to detect subtle patterns or anomalies that may be missed by human pathologists. This can lead to more precise diagnoses and better treatment decisions.
2. Personalized Medicine: Another exciting advancement is the potential for machine learning to enable personalized medicine. By analyzing vast amounts of data on patients’ genetic profiles, medical histories, and treatment outcomes, machine learning algorithms can identify specific biomarkers or genetic variations associated with different diseases. This knowledge can then be used to develop tailored treatments that are more effective and have fewer side effects.
3. Predictive Analytics: Machine learning also has the ability to predict disease progression and identify individuals at a higher risk of developing certain conditions. By continuously monitoring patient data over time, machine learning algorithms can learn from patterns and make predictions about future health outcomes. This information could help healthcare providers intervene earlier with preventive measures or targeted interventions.
4. Automation of Routine Tasks: As machine learning continues to evolve, there is a possibility for automation of routine tasks in pathological analysis such as sample preparation or image classification.
This would free up valuable time for pathologists to focus on more complex cases or collaborate with other healthcare professionals.
5 . Integration with Telemedicine: With advancements in telemedicine technology, there is an opportunity for machine learning algorithms to support remote diagnosis by providing real-time analysis of medical images or assisting pathologists during virtual consultations.
The combination of artificial intelligence (AI) technologies like natural language processing (NLP) could further enhance this collaboration between humans experts.
Ethical considerations and concerns surrounding the use of machine learning in healthcare
As with any technological advancement, the integration of machine learning in healthcare comes with its own set of ethical considerations and concerns. One major concern revolves around patient privacy and data security. With machine learning algorithms analyzing vast amounts of sensitive patient information, there is a risk that this data could be compromised or accessed without proper consent.
Another ethical consideration is the potential for bias within machine learning models. If these models are trained on datasets that are not representative or inclusive enough, they may perpetuate existing biases within healthcare systems. This could lead to unequal treatment or misdiagnosis based on factors such as race, gender, or socioeconomic status.
There is also the question of accountability when it comes to decisions made by machine learning algorithms. Who should be held responsible if an algorithm makes a mistake? Should it be the developer who created the model or the healthcare provider who implemented it? These questions raise important issues regarding transparency and liability.
There is concern over the potential job displacement of human pathologists due to automation through machine learning technologies. While these advancements can greatly improve efficiency and accuracy in pathology analysis, it raises questions about job security for those currently working in this field.
It is essential that regulatory bodies work hand-in-hand with industry experts to develop comprehensive frameworks that address these ethical considerations while harnessing the full potential of machine learning technology in pathology analysis. By doing so responsibly and thoughtfully we can reap substantial benefits while safeguarding patient rights and ensuring equitable healthcare outcomes for all individuals.
The role of human pathologists and the future of pathology
The role of human pathologists in the field of pathology remains crucial, even in the era of machine learning. While machine learning algorithms have shown great potential in aiding and enhancing pathological analysis, they cannot replace the expertise and intuition of trained professionals.
Human pathologists possess a deep understanding of disease processes, clinical context, and patient history that cannot be replicated by machines alone. They play a vital role in not only interpreting and validating the results generated by machine learning algorithms but also providing critical insights based on their experience.
Human pathologists are responsible for communicating complex findings to other healthcare providers and patients. They offer personalized care by considering individual factors that may impact diagnosis or treatment decisions. The integration of machine learning into pathology practice can amplify their capabilities rather than render them obsolete.
Looking ahead, the future of pathology is likely to see an increasing collaboration between human pathologists and machine learning technology. This synergy will enable more accurate diagnoses, quicker turnaround times, improved prediction models, and enhanced patient outcomes.
By embracing this new paradigm while emphasizing collaboration between humans and machines within strict ethical frameworks – we can harness the full potential of machine learning for pathological analysis while upholding professional standards unique to human expertise. Together with advanced technologies like AI-powered analytics tools – we can revolutionize how diseases are diagnosed leading us towards a healthier future!