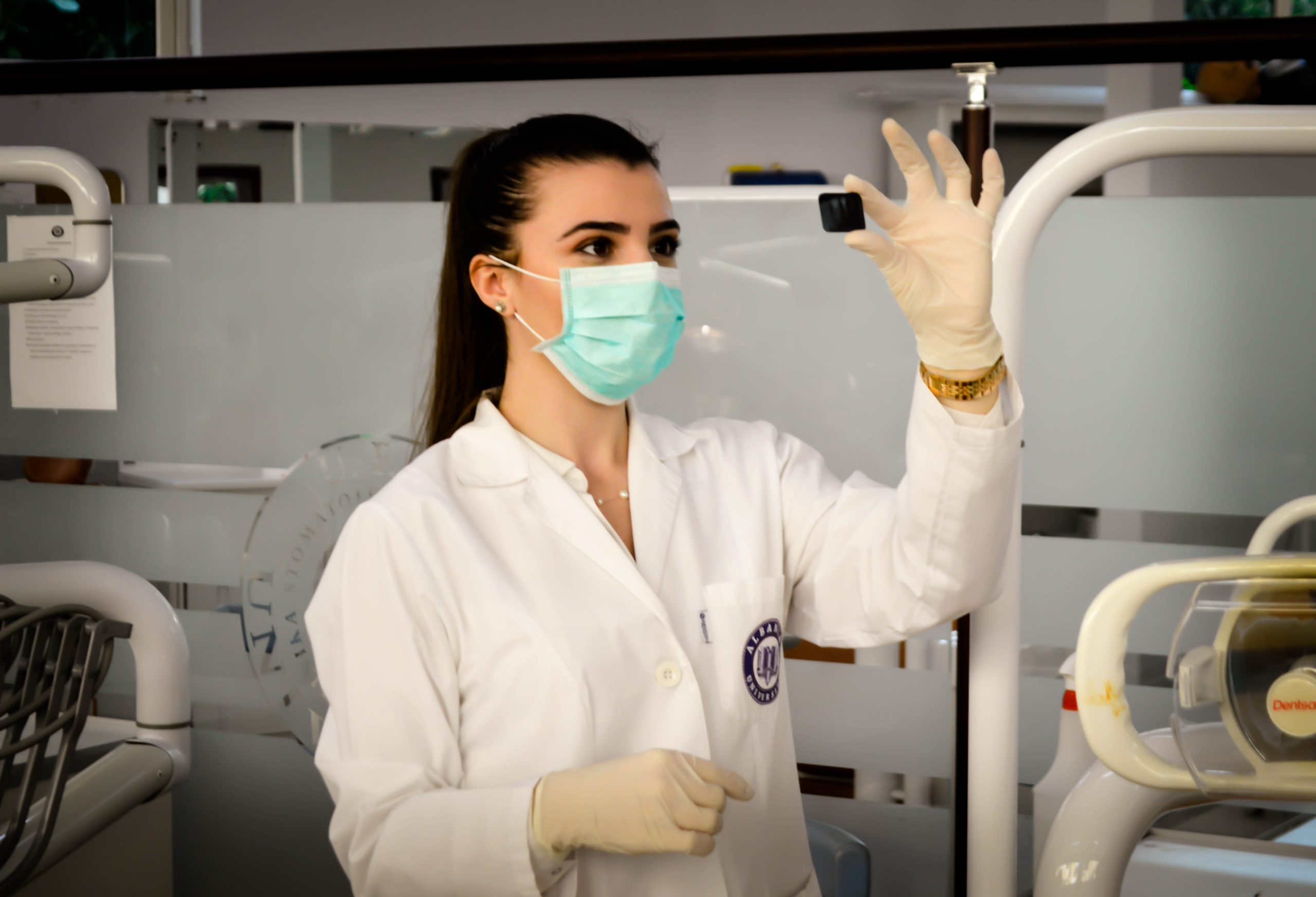
Welcome to the exciting world of machine learning and its application in the field of drug metabolism and pharmacokinetics! In today’s rapidly advancing technological landscape, machine learning has emerged as a game-changer, revolutionizing various industries. And when it comes to the pharmaceutical industry, this powerful tool holds immense potential for transforming drug development processes.
Imagine a future where medications are tailored specifically to an individual’s unique needs, minimizing side effects and maximizing therapeutic benefits. Thanks to machine learning algorithms, this vision is not far-fetched. By analyzing vast amounts of data with incredible speed and accuracy, machine learning can unravel complex patterns in drug metabolism and pharmacokinetics that were once beyond human comprehension.
We will delve into how machine learning is reshaping our understanding of drug metabolism and pharmacokinetics. We will explore its role in accelerating the drug development process while improving efficacy and safety profiles. Furthermore, we’ll discuss both the advantages and challenges associated with implementing machine learning in pharmacokinetic studies. So buckle up as we embark on an enlightening journey through the intersection of artificial intelligence and pharmaceutical science!
Understanding Drug Metabolism and Pharmacokinetics
Before diving into the role of machine learning in drug development, it’s important to have a basic understanding of drug metabolism and pharmacokinetics. These two terms are often used interchangeably but refer to different aspects of how drugs interact with the body.
Drug metabolism involves the chemical alterations that occur within the body to convert a drug into its metabolites. This process is primarily carried out by enzymes in the liver, although other organs such as the kidneys and intestines also play a role. The goal of drug metabolism is to make drugs more water-soluble so they can be easily eliminated from the body.
On the other hand, pharmacokinetics focuses on how drugs are absorbed, distributed, metabolized, and excreted by the body. It takes into account factors such as absorption rates, distribution throughout various tissues/organs, elimination half-life, and clearance rate.
Having an understanding of these processes is crucial for developing safe and effective medications. By studying how drugs are metabolized and their pharmacokinetic profiles, researchers can gain insights into factors that affect efficacy and potential adverse effects.
Now that we have covered some basics about drug metabolism and pharmacokinetics let’s explore how machine learning is revolutionizing this field!
The Role of Machine Learning in Drug Development
Machine learning has revolutionized various industries, and the field of drug development is no exception. With its ability to analyze vast amounts of data and identify patterns, machine learning has become an invaluable tool in accelerating the drug discovery process.
One key role of machine learning in drug development is in predicting the pharmacokinetics (PK) properties of potential drugs. PK refers to how a drug moves through the body, including absorption, distribution, metabolism, and excretion. By analyzing chemical structures and other relevant data, machine learning algorithms can predict important PK parameters such as clearance rates or half-life.
In addition to predicting PK properties, machine learning can also help optimize dosage regimens for specific patient populations. By analyzing patient characteristics and genetic information alongside PK data, algorithms can provide personalized dosing recommendations that maximize efficacy while minimizing side effects.
Machine learning can aid in identifying potential drug-drug interactions by mining large databases of known interactions. This helps minimize risks associated with co-administration of multiple medications.
Another crucial role of machine learning is in optimizing lead identification and optimization processes. Machine learning models can analyze vast libraries of compounds to identify those with high potential for therapeutic activity while considering factors such as safety profiles and patentability.
Machine learning techniques enable efficient analysis of complex biological networks involved in disease processes. This allows researchers to better understand disease mechanisms at a molecular level and design targeted therapies accordingly.
By streamlining various aspects of drug development – from target identification to clinical trial design – machine learning holds great promise for reducing costs and timeframes associated with bringing new drugs to market. However, it’s important to note that challenges remain regarding data quality assurance and regulatory acceptance when implementing these technologies on a larger scale.
Advantages and Challenges of Implementing Machine Learning in Pharmacokinetics
Machine learning has the potential to revolutionize the field of pharmacokinetics by enhancing our understanding of drug metabolism and optimizing drug development. One major advantage is its ability to analyze vast amounts of data quickly and accurately, leading to more precise predictions and personalized medicine.
By using machine learning algorithms, researchers can identify patterns in large datasets that may have been overlooked through traditional methods. This allows for a deeper understanding of how drugs are metabolized in the body, including factors such as absorption, distribution, metabolism, and excretion.
Another advantage is the ability to predict drug-drug interactions (DDIs) more effectively. DDIs occur when two or more drugs interact with each other in a way that alters their effectiveness or safety. Machine learning algorithms can analyze multiple variables simultaneously to identify potential DDIs before they occur, minimizing adverse effects on patients.
Although machine learning offers promising advantages for pharmacokinetic research, there are also challenges that need to be addressed. One challenge is ensuring the quality and accuracy of input data. The reliability and completeness of data sources are crucial for training accurate models.
Interpreting results generated by machine learning algorithms can be complex due to their black-box nature. It is important for researchers to understand how these models arrive at their predictions so that decisions based on these predictions can be made confidently.
Real-life Applications of Machine Learning in Drug Metabolism and Pharmacokinetics
Machine learning has shown tremendous potential in revolutionizing drug development, particularly in the field of drug metabolism and pharmacokinetics (DMPK). By leveraging large datasets and complex algorithms, machine learning techniques can help researchers gain valuable insights into how drugs are metabolized and distributed within the body.
One area where machine learning has proven invaluable is in predicting drug interactions. By analyzing vast amounts of data on known drug-drug interactions, machine learning algorithms can identify patterns and predict potential interactions between different medications. This knowledge is crucial for ensuring patient safety by avoiding harmful combinations that could lead to adverse effects or reduced efficacy.
Another important application is dose optimization. Machine learning models can analyze a wide range of factors such as patient characteristics, genetic information, and disease progression to determine the optimal dosage for an individual. This personalized approach not only improves treatment outcomes but also minimizes side effects by tailoring the medication regimen to each patient’s specific needs.
Machine learning plays a significant role in predicting drug toxicity. Traditional methods rely heavily on animal testing, which is time-consuming, expensive, and often fails to accurately predict human responses. Machine learning algorithms trained on extensive toxicological databases enable scientists to identify potential toxicities early on during the drug development process.
Machine learning assists in virtual screening processes by rapidly assessing thousands of compounds for their suitability as potential drugs based on their physicochemical properties and predicted activity against target molecules or pathways. This accelerates the identification of promising candidates for further investigation.
Machine learning also aids in optimizing clinical trial design by analyzing previous trial data to identify factors that affect success rates or predict patient response variability. By incorporating these insights into trial protocols early on, researchers can improve study designs and increase efficiency while reducing costs.
Future Possibilities and Impact of Machine Learning on the Pharmaceutical Industry
Machine learning has already made significant advancements in drug development, but its potential in the pharmaceutical industry is far from being fully realized. As technology continues to advance and datasets become more comprehensive, machine learning algorithms have the potential to revolutionize drug metabolism and pharmacokinetics.
One area where machine learning shows great promise is in predicting drug-drug interactions. By analyzing vast amounts of data from clinical trials, electronic health records, and other sources, machine learning algorithms can identify patterns that may indicate potential interactions between drugs. This information can help researchers design safer combinations of medications or warn against potentially harmful interactions.
Machine learning can also be used to optimize dosing regimens for individual patients. By considering factors such as age, weight, genetics, and other relevant variables, machine learning algorithms can develop personalized dosage recommendations that maximize therapeutic efficacy while minimizing side effects.
Another exciting possibility is using machine learning to predict a patient’s response to a particular medication. By analyzing genetic data and other biomarkers alongside clinical outcomes data, machine learning models could potentially identify which patients are most likely to respond positively or negatively to a specific treatment. This information could help clinicians make more informed decisions about which drugs would be most effective for their patients.
With the advent of wearable devices and continuous monitoring technologies, there is an opportunity to integrate real-time patient data into machine learning models. This would enable healthcare providers to monitor medication adherence and adjust treatment plans accordingly based on dynamic physiological measurements – all thanks to the power of AI-driven analytics.
Conclusion
Machine learning has emerged as a powerful tool in drug metabolism and pharmacokinetics, revolutionizing the way pharmaceutical companies approach drug development. Through its ability to analyze vast amounts of data and identify patterns, machine learning holds great promise for enhancing the efficiency and accuracy of predicting drug behavior in the human body.
By understanding how drugs are metabolized and their pharmacokinetic properties, researchers can make more informed decisions about dosage regimens, potential side effects, and overall efficacy. Machine learning algorithms can process large datasets from clinical trials, genetic information, and other sources to uncover hidden relationships that traditional methods may miss.
The advantages of implementing machine learning in pharmacokinetics are significant. It allows for faster identification of promising drug candidates while reducing costs associated with failed experiments. Additionally, it enables personalized medicine by tailoring treatments based on individual patient characteristics.
Looking ahead, the future possibilities for machine learning in pharmaceutical research are exciting. Integration with emerging technologies such as artificial intelligence (AI) could lead to even more sophisticated models that consider complex interactions between drugs and biological systems at an unprecedented level.