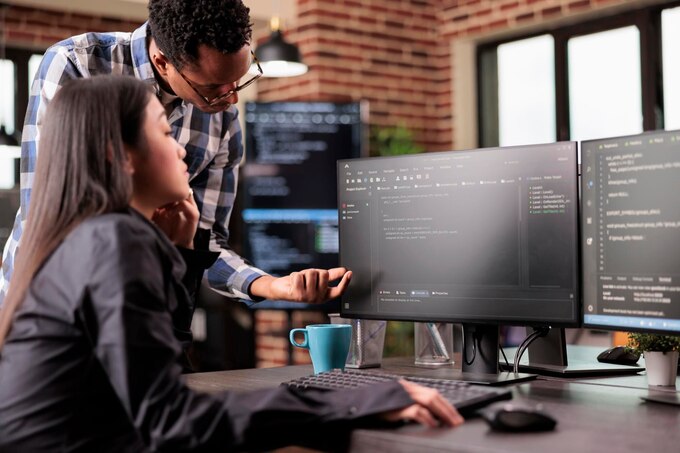
In the realm of medical imaging, every pixel tells a story. As technology evolves, so does our ability to interpret these intricate narratives. Enter machine learning—a revolutionary force making waves in pulmonary imaging.
Imagine a world where lung diseases are diagnosed with unparalleled accuracy and speed, saving lives before symptoms even appear. This is not just a dream; it’s becoming a reality thanks to rapid advancements in artificial intelligence (AI). With algorithms capable of analyzing thousands of images in mere seconds, we stand on the brink of transforming how we understand and tackle respiratory conditions.
Join us as we explore this exciting intersection of healthcare and technology. Discover how machine learning is reshaping pulmonary imaging and paving the way for groundbreaking changes in patient care.
Advancements in Pulmonary Imaging Techniques
Pulmonary imaging has experienced remarkable advancements, transforming how we visualize and understand lung health. Traditional methods like X-rays and CT scans are now complemented by innovative technologies that enhance diagnostic accuracy.
One such advancement is the integration of high-resolution imaging. This allows clinicians to observe fine details in lung structures. The clarity gained from these techniques leads to better detection of abnormalities.
Moreover, functional imaging techniques are gaining traction. These methods assess not just structural changes but also how well lungs work in real-time. By evaluating blood flow and oxygen uptake, doctors can make more informed decisions about patient care.
The evolution of artificial intelligence further enriches pulmonary imaging. AI algorithms analyze vast amounts of data quickly, identifying patterns that may elude human observation. This synergy between technology and medicine heralds a new era for diagnosing respiratory conditions effectively.
The Role of Machine Learning in Diagnosing Lung Diseases
Machine learning is transforming the landscape of lung disease diagnosis. Traditional methods often rely on human interpretation, which can be subjective and time-consuming. Machine learning algorithms analyze vast amounts of imaging data quickly and efficiently.
These algorithms excel at identifying patterns that may not be obvious to the naked eye. For instance, they can detect subtle changes in lung nodules or differentiate between types of pneumonia with remarkable accuracy.
Moreover, machine learning models continuously improve through exposure to new data. This adaptability allows for more precise diagnoses over time, reducing misdiagnosis rates and enhancing patient care.
The integration of machine learning into diagnostic workflows also streamlines processes. Radiologists are empowered to focus on complex cases while relying on AI tools for routine assessments. This collaboration could lead to faster treatment decisions and improved outcomes for patients battling lung diseases.
Benefits of Using Machine Learning in Pulmonary Imaging
Machine learning is transforming pulmonary imaging, offering numerous advantages for healthcare professionals. One of the most significant benefits is enhanced accuracy in diagnosing lung diseases. With sophisticated algorithms, machine learning can analyze vast datasets and identify patterns that may escape human eyes.
Additionally, it streamlines workflows in radiology departments. Automated image analysis reduces the time needed to interpret scans, allowing clinicians to focus on patient care rather than manual evaluations. Faster diagnoses lead to quicker treatment decisions, which can be critical for patients with severe conditions.
Moreover, machine learning models continuously learn from new data. This means they adapt over time and improve their predictive capabilities. As more lung images are processed, these systems become even more reliable.
Cost-effectiveness also plays a role; by minimizing errors and optimizing resource use within hospitals, machine learning could reduce overall healthcare expenses while enhancing patient outcomes across the board.
Challenges and Limitations of Implementing Machine Learning in Pulmonary Imaging
Implementing machine learning in pulmonary imaging comes with its own set of challenges. One major hurdle is the need for high-quality, annotated datasets. Many existing datasets are small or not diverse enough, limiting the effectiveness of algorithms.
Another issue is interpretability. Clinicians often find it difficult to understand how a model arrives at its conclusions. This lack of transparency can hinder trust and adoption among healthcare professionals.
Regulatory concerns also pose significant obstacles. The medical field requires strict adherence to guidelines, which may slow down the integration of innovative technologies.
Furthermore, there’s the challenge of integrating these systems into existing workflows. Training staff and ensuring interoperability with current imaging equipment takes time and resources that many facilities struggle to allocate.
Ethical considerations regarding patient data privacy must be navigated carefully as we embrace advancements in this area.
Conclusion
The integration of machine learning into pulmonary imaging represents a transformative shift in how lung diseases are diagnosed and managed. As technology advances, we see clearer images, faster diagnostics, and improved patient outcomes. Machine learning algorithms can analyze vast amounts of data more quickly than any human could, identifying patterns that may go unnoticed.
However, challenges remain. Data privacy concerns and the need for robust training datasets must be addressed to ensure reliable results. The accuracy of these models depends significantly on the quality of input data.
Despite these hurdles, the potential benefits are enormous. Enhanced diagnostic capabilities combined with rapid analysis can lead to early detection and treatment of serious lung conditions. This progress not only improves individual care but also has implications for public health as a whole.
As researchers continue to explore this promising intersection between technology and medicine, the future looks bright for pulmonary imaging influenced by machine learning innovations. There’s much anticipation surrounding what lies ahead in this field—an exciting journey is unfolding.